Unlock Data Potential with Box Cox Power Transformation

<!DOCTYPE html>
In the world of data analysis, transforming variables is a crucial step to meet the assumptions of statistical models. One powerful technique that stands out is the Box Cox Power Transformation. Whether you're dealing with skewed data or non-normal distributions, this method can help normalize your data, making it suitable for a wide range of analyses. By understanding and applying the Box Cox transformation, you can unlock the full potential of your data, leading to more accurate and reliable results. (data transformation, statistical analysis, skewed data)
What is the Box Cox Power Transformation?

The Box Cox Power Transformation is a statistical technique used to transform non-normal data into a normal distribution. Developed by George Box and David Cox in 1964, it involves applying a power transformation to the data based on a parameter lambda (λ). The transformation is defined as:
For λ ≠ 0: ( y_i^{(\lambda)} = \frac{y_i^\lambda - 1}{\lambda} )
For λ = 0: ( y_i^{(0)} = \log(y_i) )
The optimal value of λ is typically determined using maximum likelihood estimation, ensuring the transformed data closely resembles a normal distribution. (Box Cox transformation, normal distribution, lambda parameter)
Why Use Box Cox Transformation?
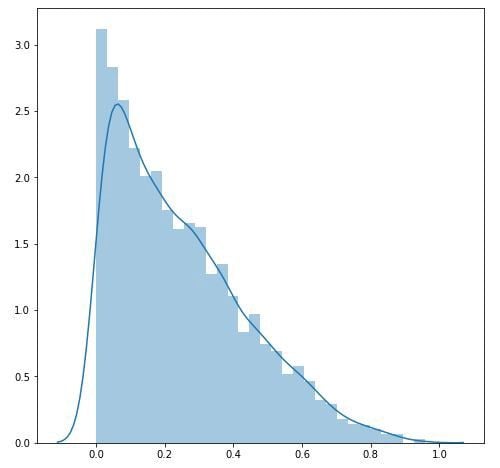
The Box Cox transformation is particularly useful in scenarios where data exhibits skewness or violates the assumptions of parametric tests. Here are some key benefits:
- Normalizes Data: Transforms skewed data into a more normal shape.
- Improves Model Fit: Enhances the performance of linear regression and other models.
- Simplifies Analysis: Makes data easier to interpret and analyze.
By addressing these issues, the Box Cox transformation ensures that your statistical analyses are robust and reliable. (data normalization, model fit, statistical robustness)
How to Apply Box Cox Transformation

Applying the Box Cox transformation involves several steps. Here’s a concise guide:
- Explore Data: Visualize your data using histograms or Q-Q plots to identify skewness.
- Estimate Lambda: Use statistical software to find the optimal λ value.
- Transform Data: Apply the transformation using the estimated λ.
- Verify Results: Check the transformed data for normality using tests like Shapiro-Wilk.
📊 Note: Always validate the transformation’s effectiveness before proceeding with further analysis.
(data exploration, lambda estimation, transformation verification)
Box Cox Transformation in Practice

The Box Cox transformation is widely used across various fields, including finance, healthcare, and engineering. For instance, in financial analysis, it can normalize stock returns for better risk assessment. In healthcare, it helps standardize patient data for predictive modeling. Below is a comparison of its applications:
Field | Application |
---|---|
Finance | Normalizing stock returns |
Healthcare | Standardizing patient data |
Engineering | Analyzing material strength |
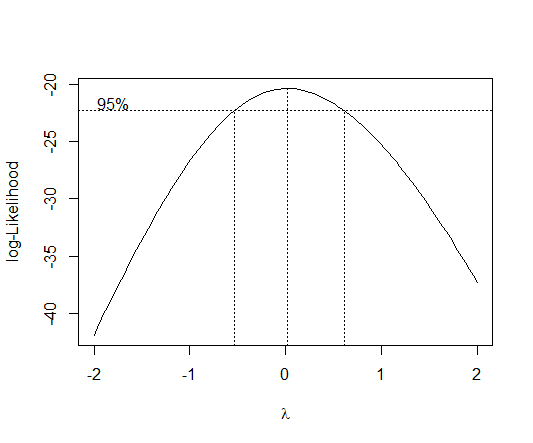
(financial analysis, healthcare data, engineering applications)
Checklist for Box Cox Transformation

To ensure a successful transformation, follow this checklist:
- Inspect data for skewness.
- Estimate the optimal λ value.
- Apply the transformation.
- Verify normality of transformed data.
- Document the process for reproducibility.
(transformation checklist, data inspection, process documentation)
The Box Cox Power Transformation is a versatile tool that can significantly enhance your data analysis capabilities. By normalizing skewed data and improving model fit, it ensures that your statistical conclusions are both accurate and reliable. Whether you're a data scientist, researcher, or analyst, mastering this technique will undoubtedly elevate your data-driven decision-making. (data analysis, statistical conclusions, decision-making)
What is the Box Cox Transformation used for?
+The Box Cox Transformation is used to normalize skewed data, making it suitable for statistical analyses that assume normality.
How do I determine the optimal lambda value?
+The optimal lambda value is typically determined using maximum likelihood estimation, often performed using statistical software.
Can the Box Cox Transformation handle negative data?
+The standard Box Cox Transformation cannot handle negative data. However, you can add a constant to the data to make all values positive before applying the transformation.