Policy Learning & Causal Inference: Unlocking Data-Driven Decisions

In today’s data-driven world, making informed decisions is no longer a luxury—it’s a necessity. Policy learning and causal inference are two powerful methodologies that bridge the gap between raw data and actionable insights. By understanding the causal relationships within data, organizations can predict outcomes, optimize policies, and drive impactful results. Whether you’re a data scientist, policymaker, or business leader, mastering these techniques can revolutionize your decision-making process. (Data-driven decisions, Causal inference, Policy optimization)
What is Policy Learning?

Policy learning involves using data to identify the best course of action in complex systems. It leverages machine learning and optimization techniques to evaluate and improve decision-making strategies. For instance, in healthcare, policy learning can help determine the most effective treatment plans based on patient data. (Machine learning, Decision-making strategies, Healthcare analytics)
Key Applications of Policy Learning
- Healthcare: Personalized treatment recommendations.
- Finance: Risk management and fraud detection.
- Public Policy: Resource allocation and policy impact analysis.
Why Causal Inference Matters
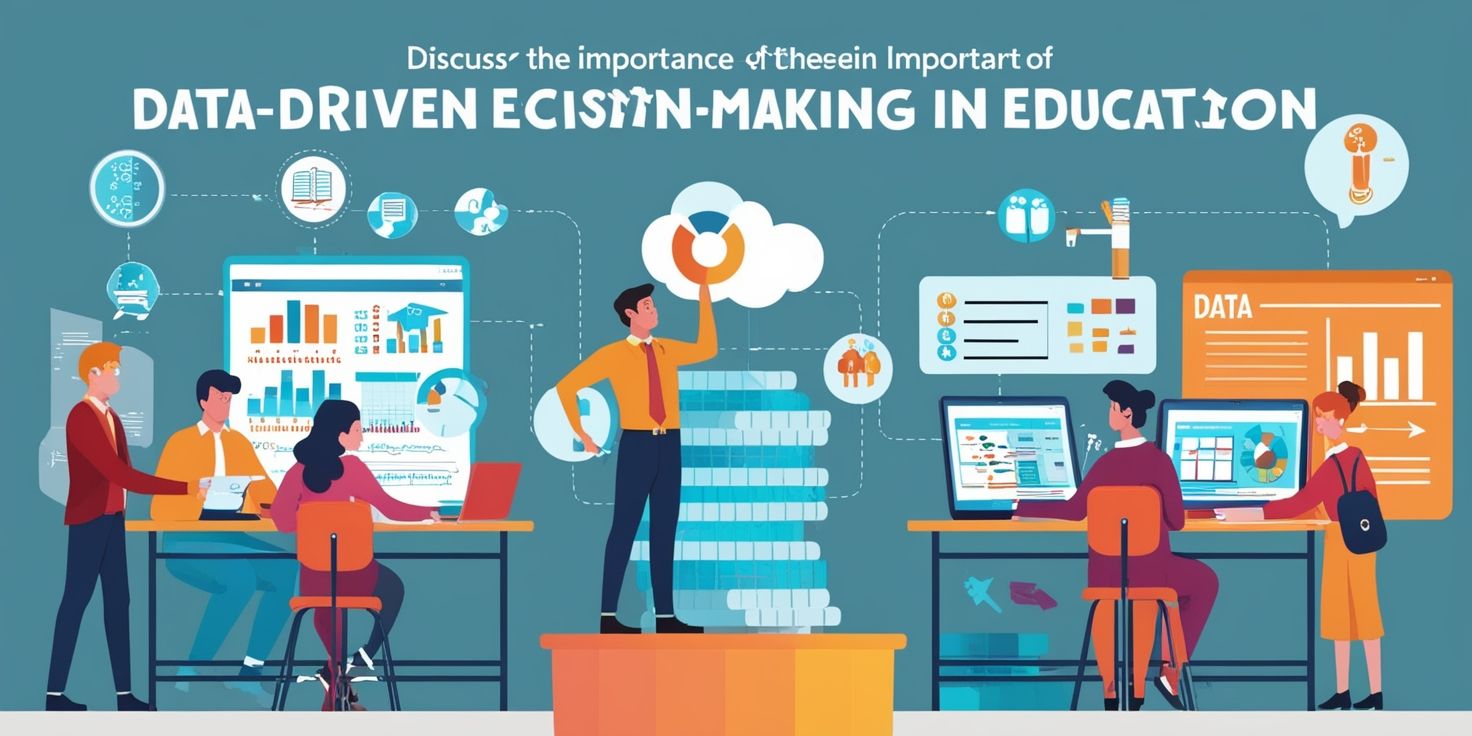
Causal inference goes beyond correlation to uncover cause-and-effect relationships in data. Unlike traditional statistical methods, it helps answer questions like, “What would happen if we implemented this policy?” This is crucial for predicting the outcomes of decisions and avoiding biases. (Causal relationships, Predictive analytics, Bias reduction)
Techniques in Causal Inference
Technique | Application |
---|---|
Propensity Score Matching | Comparing treatment and control groups. |
Instrumental Variables | Addressing unobserved confounders. |
Difference-in-Differences | Evaluating policy impacts over time. |
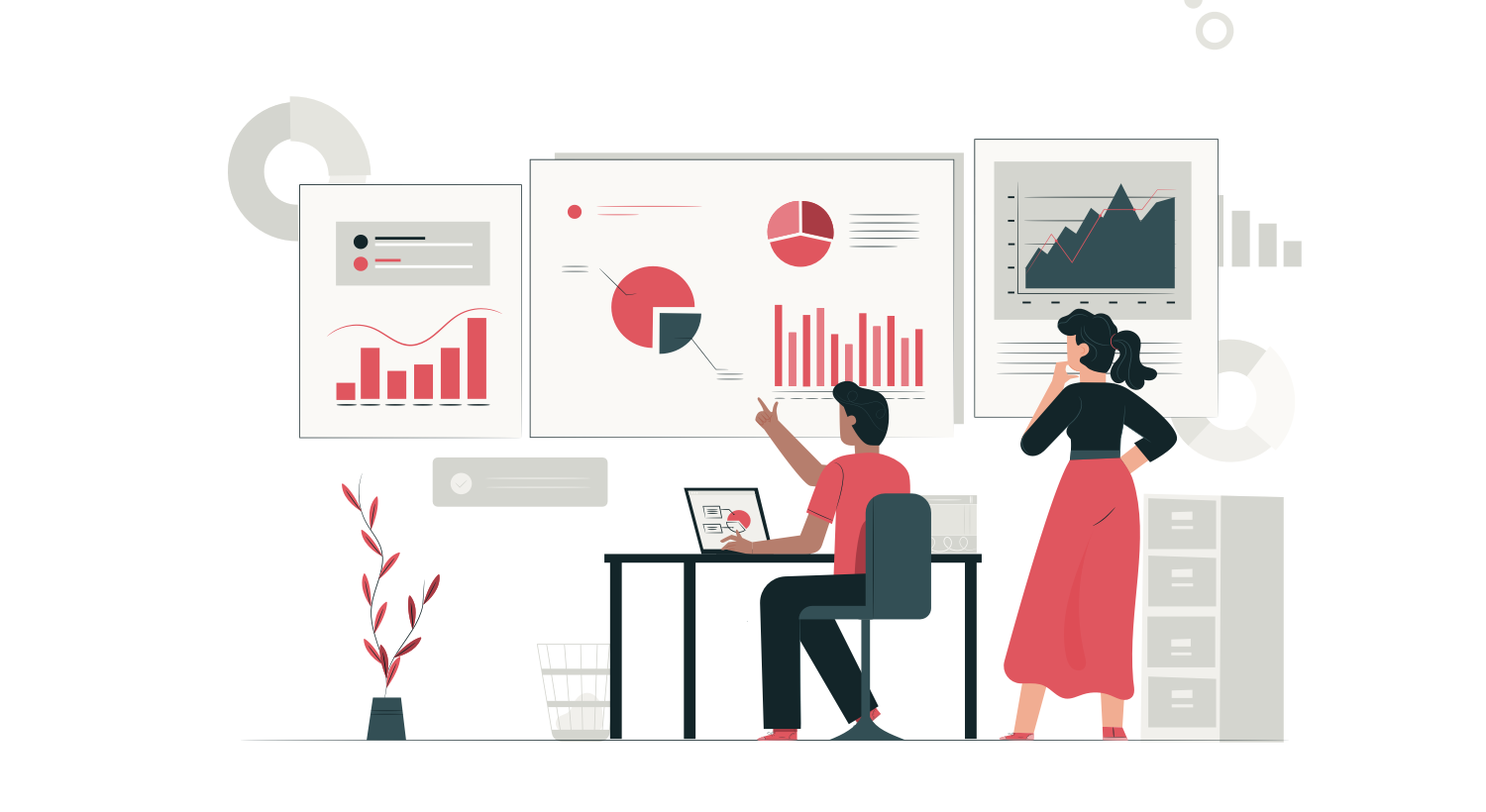
Integrating Policy Learning and Causal Inference

When combined, policy learning and causal inference create a robust framework for data-driven decision-making. For example, causal inference can identify which factors truly influence outcomes, while policy learning optimizes actions based on these insights. This synergy is particularly valuable in industries like healthcare, finance, and public policy. (Data-driven framework, Industry applications, Decision optimization)
Steps to Implement Integrated Solutions
- Data Collection: Gather relevant and high-quality data.
- Causal Analysis: Identify causal relationships using appropriate techniques.
- Policy Optimization: Use machine learning to refine decision-making strategies.
- Evaluation: Test and validate the optimized policies.
📌 Note: Ensure your data is clean and representative to avoid skewed results.
Essential Tools for Policy Learning and Causal Inference
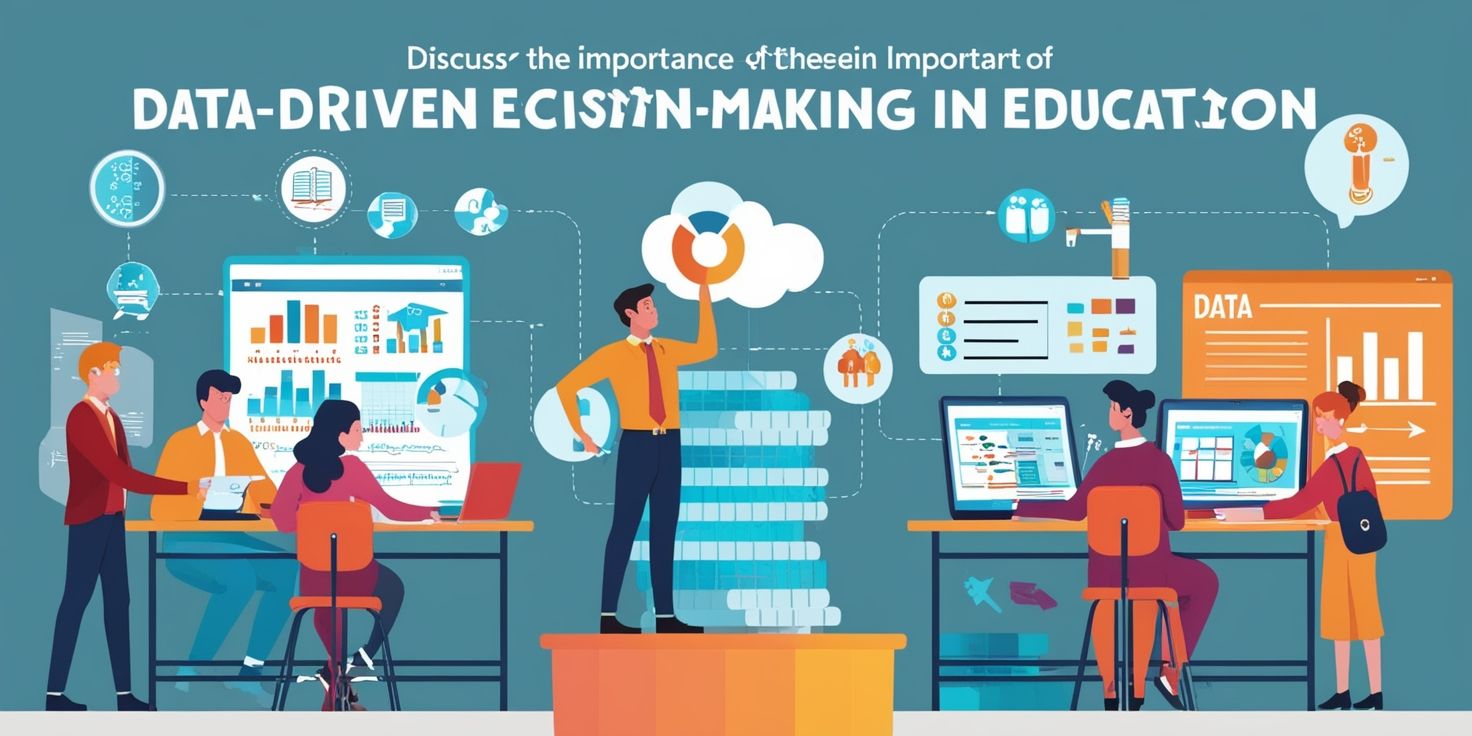
Several tools and technologies can streamline the implementation of these methodologies. Popular choices include:
- Python Libraries: CausalML, EconML, and DoWhy.
- R Packages: MatchIt, causact, and DeclareDesign.
- Platforms: Google Cloud AI, AWS SageMaker, and Microsoft Azure Machine Learning.
By harnessing the power of policy learning and causal inference, organizations can unlock the full potential of their data. These methodologies not only enhance decision-making but also pave the way for innovative solutions across industries. Whether you’re optimizing healthcare policies or improving financial strategies, the integration of these techniques is a game-changer. (Decision-making innovation, Industry solutions, Data potential)
What is the difference between policy learning and causal inference?
+
Policy learning focuses on optimizing decision-making strategies using data, while causal inference identifies cause-and-effect relationships to understand outcomes.
How can causal inference reduce bias in data analysis?
+
Causal inference uses techniques like propensity score matching and instrumental variables to account for confounding factors, ensuring more accurate results.
Which industries benefit most from policy learning?
+
Industries like healthcare, finance, and public policy benefit significantly due to their need for data-driven decision-making and resource optimization.