Randomization Test: Theory, Applications, and Insights
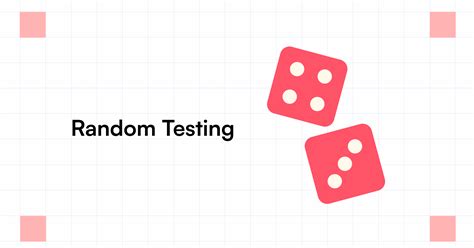
Randomization tests, also known as permutation tests, are a powerful statistical tool used to assess the significance of observed data without relying on strict assumptions about the underlying distribution. Unlike traditional parametric tests, randomization tests are non-parametric, making them versatile and robust in various applications. This blog explores the theory, applications, and insights of randomization tests, providing a comprehensive guide for both informational and commercial audiences. (randomization test, statistical significance, non-parametric tests)
Understanding the Theory of Randomization Tests
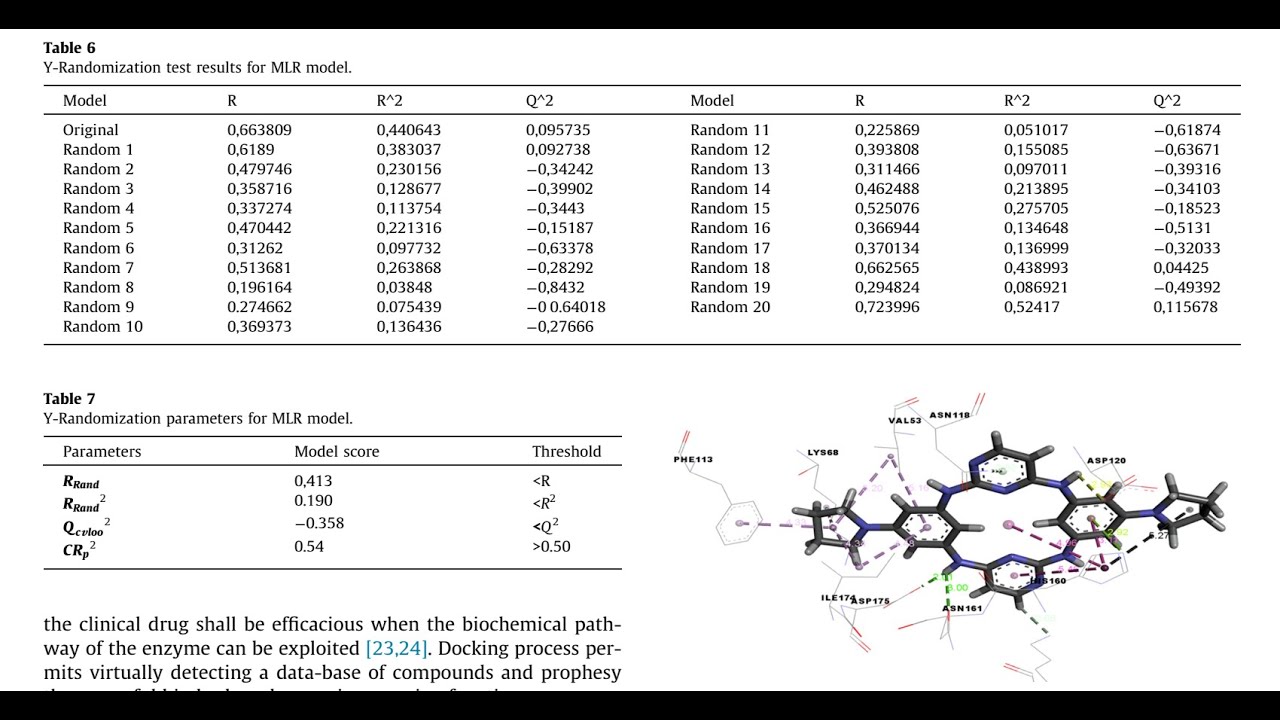
Randomization tests work by reshuffling the data to simulate the null hypothesis. By comparing the observed outcome to the distribution of outcomes under random rearrangements, we can determine the likelihood of observing the data by chance alone. This method is particularly useful when sample sizes are small or data distributions are unknown.
Key Concepts in Randomization Tests
- Null Hypothesis: Assumes no relationship between variables.
- Permutation: Rearranging data to generate all possible outcomes.
- P-value: The probability of observing the data under the null hypothesis.
📌 Note: Randomization tests are especially valuable when traditional tests like t-tests or ANOVA are not applicable due to data constraints.
Applications of Randomization Tests

Randomization tests are widely used across various fields, including biology, economics, and machine learning. Below are some key applications:
1. A/B Testing in Marketing
Marketers use randomization tests to compare the effectiveness of two versions of a campaign. By randomly assigning participants to groups, they can determine if differences in outcomes are statistically significant.
2. Clinical Trials in Healthcare
In medical research, randomization tests help evaluate the efficacy of treatments by comparing patient outcomes across randomized groups.
3. Quality Control in Manufacturing
Manufacturers use these tests to identify defects in production processes by comparing sample data to expected outcomes.
Field | Application |
---|---|
Marketing | A/B Testing |
Healthcare | Clinical Trials |
Manufacturing | Quality Control |

Insights and Best Practices
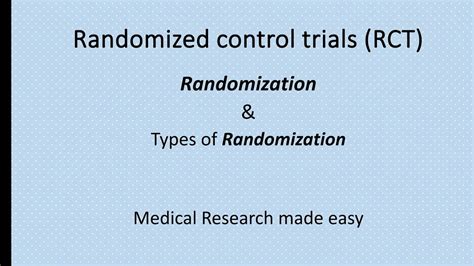
To effectively implement randomization tests, consider the following insights:
- Choose the Right Test: Ensure the test aligns with your research question and data type.
- Sample Size Matters: While randomization tests are robust, larger sample sizes improve accuracy.
- Compute Power: These tests can be computationally intensive, especially with large datasets.
💡 Note: Always validate results with multiple permutations to ensure reliability.
Checklist for Implementing Randomization Tests

- Define the null hypothesis clearly.
- Randomly permute the data to generate test statistics.
- Calculate the p-value by comparing the observed statistic to the permutation distribution.
- Interpret results in the context of your research question.
Randomization tests offer a flexible and reliable approach to statistical inference, making them an essential tool for data analysis. Whether you’re a researcher, marketer, or quality control specialist, understanding and applying these tests can enhance your decision-making process. By focusing on the theory, applications, and best practices, you can leverage randomization tests to uncover meaningful insights from your data. (statistical inference, data analysis, decision-making)
What is a randomization test?
+
A randomization test is a non-parametric statistical method that assesses the significance of observed data by reshuffling it to simulate the null hypothesis.
When should I use a randomization test?
+
Use it when traditional parametric tests are not applicable due to small sample sizes or unknown data distributions.
How does a randomization test differ from a t-test?
+
Unlike t-tests, randomization tests do not assume normality or equal variances, making them more flexible for diverse datasets.