Explanatory Variable Stats: Understanding Key Drivers in Data Analysis
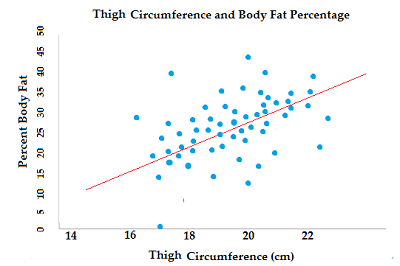
In the world of data analysis, understanding the explanatory variables that drive your results is crucial. These variables, also known as independent variables, are the factors you manipulate or observe to determine their impact on the dependent variable. Whether you’re a data scientist, marketer, or business analyst, grasping the concept of explanatory variable stats can significantly enhance your ability to draw meaningful insights from data. This post will delve into the importance of explanatory variables, how to identify them, and their role in various analytical techniques, ensuring you can leverage them effectively in your projects. (explanatory variable stats, data analysis, independent variables)
What Are Explanatory Variables?

Explanatory variables are the inputs in a statistical model that help explain changes in the response variable (dependent variable). For instance, in a study on sales performance, factors like advertising spend, seasonality, and product pricing could be explanatory variables. Understanding these variables allows analysts to uncover patterns, make predictions, and inform decision-making. (explanatory variable stats, response variable, statistical model)
Key Characteristics of Explanatory Variables
- Relevance: They must be directly related to the outcome being studied.
- Measurability: They should be quantifiable or categorizable for analysis.
- Independence: Ideally, they should not be highly correlated with each other to avoid multicollinearity.
📊 Note: Always ensure your explanatory variables are relevant and measurable to avoid misleading conclusions.
How to Identify Explanatory Variables

Identifying the right explanatory variables is a critical step in data analysis. Here’s a structured approach:
- Define the Problem: Clearly outline the question or hypothesis you’re addressing.
- Review Literature: Look for existing studies to identify commonly used variables.
- Data Exploration: Analyze your dataset to uncover potential relationships.
- Expert Input: Consult domain experts for insights into relevant factors.
Step | Description |
---|---|
1 | Define the Problem |
2 | Review Literature |
3 | Data Exploration |
4 | Expert Input |

Common Mistakes to Avoid
- Overlooking Variables: Failing to consider all potential drivers.
- Including Irrelevant Variables: Adding variables that don’t impact the outcome.
- Ignoring Multicollinearity: Using highly correlated variables, which can skew results.
Role of Explanatory Variables in Analytical Techniques

Explanatory variables are integral to various analytical methods, including regression analysis, machine learning models, and hypothesis testing. In regression, they help quantify the relationship between predictors and the outcome. In machine learning, they serve as features to train models. (regression analysis, machine learning, hypothesis testing)
Regression Analysis
In linear regression, explanatory variables are used to predict the dependent variable. For example, predicting house prices based on size, location, and age.
Machine Learning
In algorithms like decision trees or neural networks, explanatory variables act as inputs to build predictive models.
🤖 Note: Ensure your explanatory variables are cleaned and preprocessed for optimal model performance.
Checklist for Working with Explanatory Variables

- Define the problem clearly.
- Identify relevant variables through literature and data exploration.
- Check for multicollinearity and irrelevance.
- Preprocess variables for analysis.
- Validate findings with domain experts.
In summary, explanatory variables are the backbone of data analysis, enabling analysts to uncover relationships and make informed decisions. By identifying and leveraging these variables effectively, you can enhance the accuracy and reliability of your models. Whether you’re predicting sales, analyzing customer behavior, or optimizing processes, understanding explanatory variable stats is essential for success. (explanatory variable stats, data analysis, predictive models)
What is the difference between explanatory and response variables?
+
Explanatory variables (independent variables) are the factors you manipulate or observe, while the response variable (dependent variable) is the outcome you measure.
How do I avoid multicollinearity in explanatory variables?
+
Use techniques like variance inflation factor (VIF) or correlation matrices to identify and remove highly correlated variables.
Can explanatory variables be categorical?
+
Yes, explanatory variables can be categorical (e.g., gender, region) or numerical (e.g., age, income).